Feeding AI-generated content back into an AI model results in complete disorder. A new study published in Nature reveals that when AI models are trained using AI-generated data, they quickly suffer from “model collapse.” This phenomenon causes the AI’s outputs to become increasingly strange, jumbled, and nonsensical, suggesting that synthetic data can disrupt the model’s functionality more than human-generated content.
This research highlights the sensitivity of AI models to their training data and the potential risks of incorporating AI-generated material into their datasets. It also emphasizes the growing need for high-quality human-generated content, which is becoming scarcer and therefore more valuable, potentially stalling advancements in generative AI.
Zakhar Shumaylov, an AI researcher at the University of Cambridge and co-author of the study, underscores the importance of carefully curating training data. He warns that failure to do so will lead to inevitable problems.
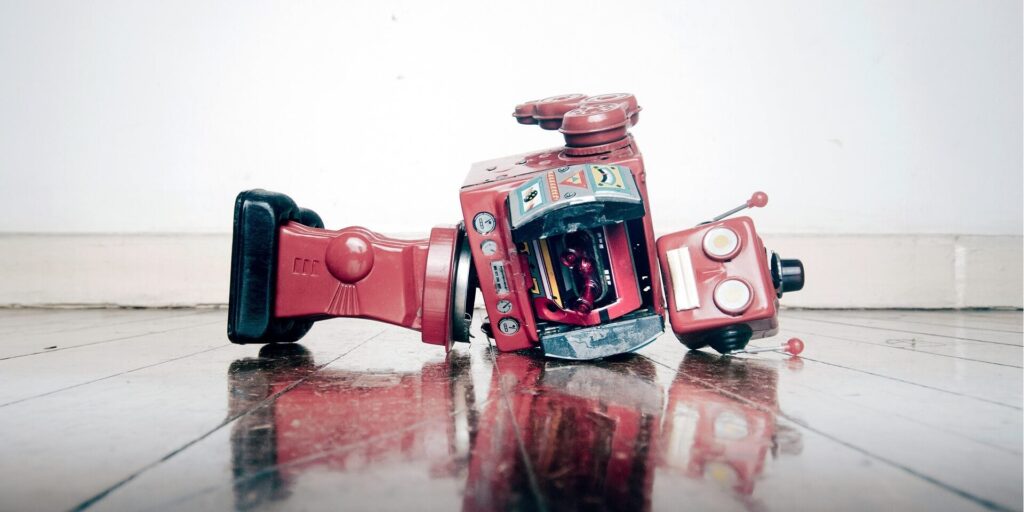
In the study, Shumaylov’s team used a pre-trained large language model (LLM) and trained it with a dataset from HuggingFace that included Wikipedia entries. They then iterated the model’s output through multiple cycles, feeding each result back into the training set. The outcomes were striking: an initial query about buildings in Somerset, England, produced relatively normal responses. However, by the ninth iteration, the output devolved into incoherent text about jackrabbit tails.
The mechanism behind model collapse is straightforward. AI systems rely solely on their training data; high-quality and diverse data lead to better-performing models, while repetitive AI-generated content limits diversity. This process causes the model to amplify its own mistakes, lose certain terms, and eventually break down.
This issue isn’t new. AI researcher Jathan Sadowski previously described a similar phenomenon as “Habsburg AI,” likening it to the inbreeding of Europe’s Habsburg family, which led to genetic decline. Just as genetic diversity is crucial for humans, diversity in training data is essential for maintaining AI models’ functionality.
The study also raises concerns about the sustainability of web scraping for data collection. As the internet becomes saturated with AI-generated content—from spammy news sites to bizarre AI-created images—web scraping is becoming a less reliable method for gathering training data. The study notes the challenge of distinguishing AI-generated content from human-generated content and questions how to track such data on a large scale.
On a positive note, the study suggests that integrating more original human data can slow down the process of model collapse. Nevertheless, AI models have a significant demand for high-quality and original data. The question remains: can AI companies meet this growing need?